Vision
As the growing worldwide population becomes more mobile and urbanized, the risks that infectious diseases epidemic and their associated threats may reach global proportions are constantly increasing. To effectively limit the social and economic damage caused by infectious diseases, the public health communities need to be in the position to anticipate the spatial and temporal evolution of epidemics and evaluate the potential impact of available containment and prevention strategies.
The global epidemic and mobility model, GLEAM, combines real-world data on populations and human mobility with elaborate stochastic models of disease transmission to deliver analytic and forecasting power to address the challenges faced in developing intervention strategies that minimize the impact of potentially devastating epidemics. You can learn more about the GLEAM Project here. A list of publications using GLEAM can be found here.
Challenges
GLEAM supports policy-making and emergency planning by developing epidemic models and scenario analysis able to gauge the actual threat of highly pathogenic diseases.
In the event of epidemic outbreaks public health authorities can choose to activate prevention and emergency response policies such as vaccination, travel restrictions, or school and business closures. The socio-economic cost of such programs can be high and their impact on the epidemic hard to determine. GLEAM allows the modeling of containment and mitigation strategies providing quantitative projections that better informs the analysis of their likely impact.
Epidemic forecasts need to consider directly transmissible infectious diseases within a social context. How many people live and work in a particular area and how much do they interact and travel? GLEAM uses real-world data covering the distribution of the world-wide population, their daily interactions and journeys, and the spatial structure and volumes of national and international air traffic. By combining these datasets with realistic models of infection dynamics, GLEAM can deliver forecasts for the spreading pattern of infectious diseases epidemics.
We have thoroughly tested and validated GLEAM against historical epidemic outbreaks including the 2002/03 SARS epidemic. In 2009 GLEAM has been used to produce real time forecast of the unfolding of the H1N1 pandemic.
In a globalized world we cannot ignore the international spread of disease. International travel will bring diseases in, and spread infections to far flung communities. Public health authorities can only prepare and plan if they know the full picture. GLEAM simulations considers this global perspective and include description capacities in countries or regions that do not have comprehensive disease monitoring programs or modeling capacities.
The GLEAM approach to global epidemic forecasting deliver models and data that can be used by policy makers to anticipate trends, evaluate risks and eventually manage future events.
Model
GLEAM integrates three layers: real-world data on the global population; real-world data on the mobility of this population; an individual based stochastic mathematical model of the infection dynamics.
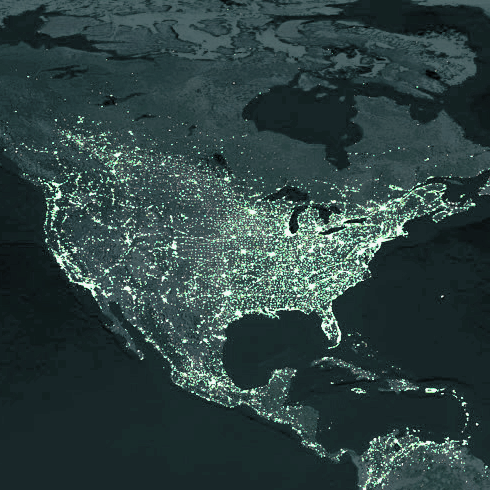
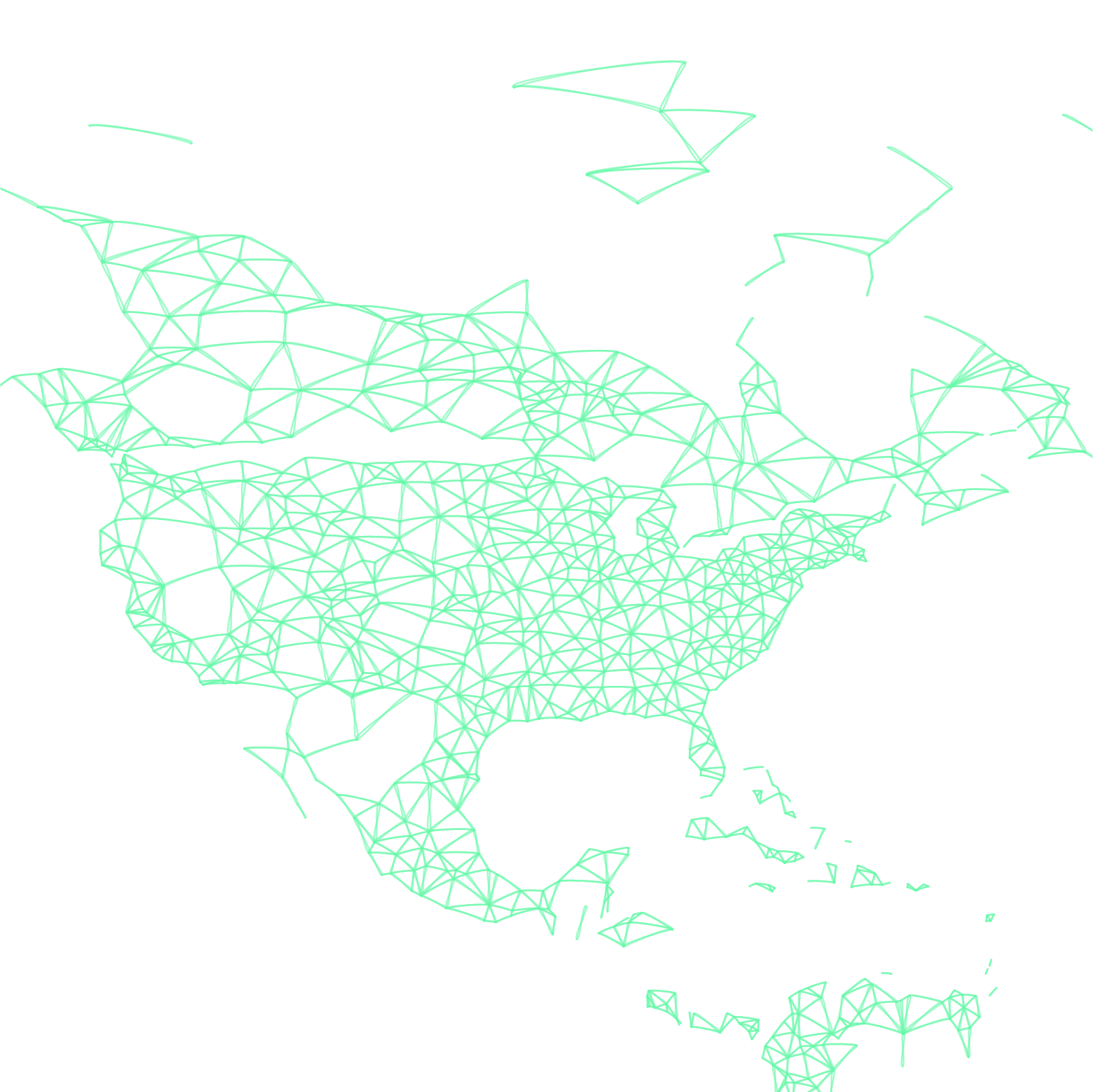
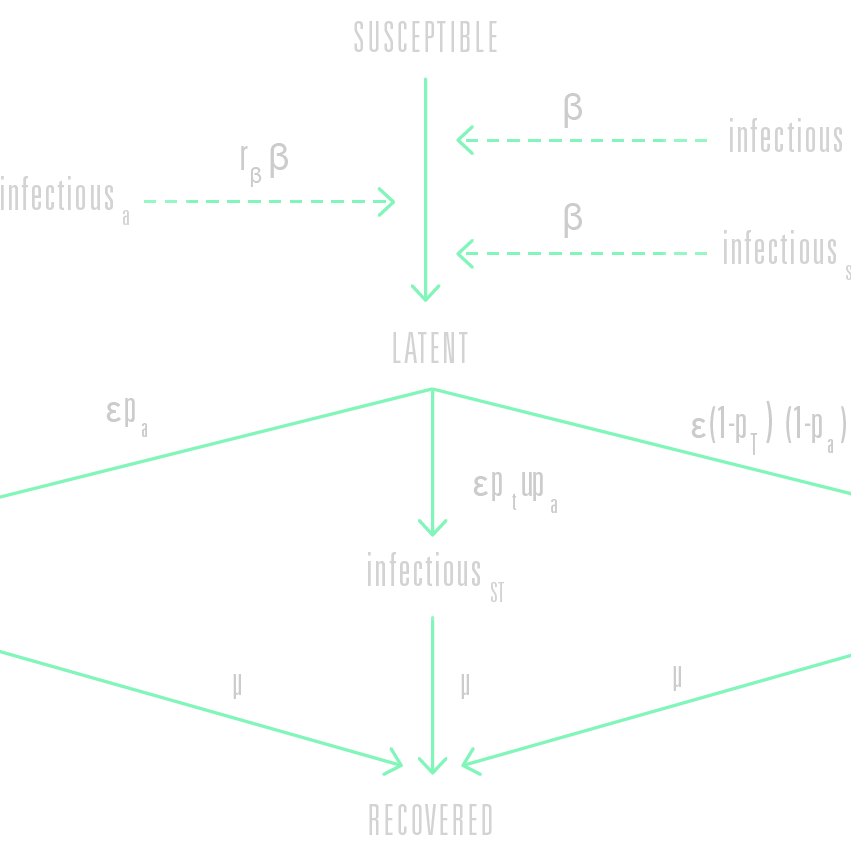
GLEAM acquires population data from the websites of the Gridded Population of the World and the Global Urban-Rural Mapping projects, which are run by the Socioeconomic Data and Application Center (SEDAC) of Columbia University.
In these datasets the world is divided into a grid of cells and assigned an estimated population value. GLEAM uses cells that are approximately 25 x 25 km, dividing the globe into over 250,000 populated cells.
We know the coordinates of each cell and also those of all the commercial airports in the World Airport Network. By considering the distance between the cells and airports we assign each cell to a ‘local’ airport; this process generates over 3,300 subpopulations, each centered on a local transportation hub.
Airport network
GLEAM uses a set of twelve different flight networks (one for each month) derived from the worldwide booking datasets from the Official Airline Guide (OAG) database. This database contains more than 3,800 commercial airports in about 230 countries, and includes over 4,000,000 connections representing the estimated bookings between any two of these airports for each month.
Commuting network
Our commuting database has been compiled using information obtained from the national statistics offices of more than 40 countries in five continents, covering more than 78,000 administrative regions. These data sources, which use different semantics and organizational structures with varying degrees of detail have been standardized before being integrated.
Our fully integrated dataset contains over five million commuting connections between GLEAM’s geographic subpopulations, capturing the irregular network structure that affects the local diffusion of infections between neighboring subpopulations.
The GLEAM engine simulates the infection dynamics according to the characteristics of the disease coupled with any prevention and intervention measures. Examples of disease characteristics are: incubation times, the proportion of asymptomatic yet infectious individuals, mortality rates and immunity.
The infection characteristics are defined in a so-called ‘compartmental model’. Each individual fits, at any given point in time, within a certain ‘compartment’ that corresponds with a particular disease-related state (being susceptible, symptomatic or vaccinated, for example). These compartments are connected by paths that define how individuals may pass from one state to another (from susceptible to latent when being infected, for example) while associated parameters determine the likelihood that such transitions take place.
GLEAM uses stochastic algorithms mathematically defined through individual based stochastic chain binomial and multinomial processes to calculate the proportion of the population within each compartment for each subpopulation, and how these proportions change over time as individuals transition from one compartment to the next.